Abstract
For years, Multi-Touch Attribution (MTA) has been the cornerstone of campaign measurement, relying on third-party cookies to track users across the web and pinpoint the impact of each touchpoint on the customer journey. However, with the growing walled gardens limiting cross-channel views since 2020 and now the gradual demise of third-party cookies, the very foundation of traditional attribution models has been shaken. These developments have prompted a dramatic transformation in the realm of attribution, leading to innovative approaches using Artificial Intelligence (AI), data science and new technological platforms. Companies that effectively leverage these cutting-edge tools and technology alongside valuable first-party data will emerge as leaders in the privacy-first era.
Walled Gardens Limit Data Access And Breadth Of Measurement
Walled gardens, such as Google, Meta, and Apple, have made MTA more complex. By operating as closed ecosystems, they restrict data access and sharing, making it difficult to track and optimize customer journeys across platforms. A pure e-commerce business which solely advertises on Google, can still gain some insights from such a restricted view of their advertising efforts. However, for all other, and the majority of, businesses, this fragmented landscape increases costs and challenges of trying to accurately stitch together consumer journeys and measure campaign impact across online platforms but also across offline properties – both offline sales and offline media are effectively excluded from that ecosystem. Non-media internal and external factors are also left out. In addition, different platforms employ unique attribution methodologies, making cross-platform comparisons difficult and potentially misleading.
All in all, this fragmented landscape hinders the comprehensive view of a customer's journey that marketers need to accurately measure and optimize their marketing spend.
Third-Party Cookies Will Eventually Become Obsolete
Third-party cookies have long been the backbone of digital advertising, providing a seemingly perfect solution for marketers. By following users around the web, they offered a detailed picture of how each ad, email, or social media post influenced conversions. However, this level of tracking raised privacy concerns, leading to stricter regulations and ultimately, for major browsers to phase out support for these cookies. While Apple and Mozilla have led the charge in phasing out third-party cookies and prioritizing user privacy with measures like App Tracking Transparency, Google, despite its dominant browser market share[1], has been slower to implement similar changes.
Google's July 2024 announcement to scrap or indefinitely postpone the elimination of third-party cookies generated considerable buzz within the industry but it will ultimately have little impact on where it’s headed. While this delay offers more time to transition to a cookie-less future, consumer privacy concerns and the increasing regulatory landscape continue to drive the industry towards a more privacy-centric approach. The fundamental challenge of balancing user privacy with effective advertising measurement remains. Marketers must still prioritize first-party data and explore alternative measurement solutions to ensure long-term success, and they already have been hard at work with it.
Google’s alternative to the cookie deprecation includes introducing a new one-time prompt giving users the ability to opt out of tracking as well further developing their Privacy Sandbox capabilities.
These increased privacy options alongside the ongoing challenges of cross-device tracking and user identification underscore the demand for more effective attribution methods.
Rethinking Attribution Models
The challenges posed by walled gardens along with the eventual demise of cookies don’t signal the fall of granular campaign attribution. They simply demand a shift in approach. One that has privacy preservation at its core, where consent-based or aggregated first-party data is a key input to advanced attribution methods powered by Machine Learning and AI.
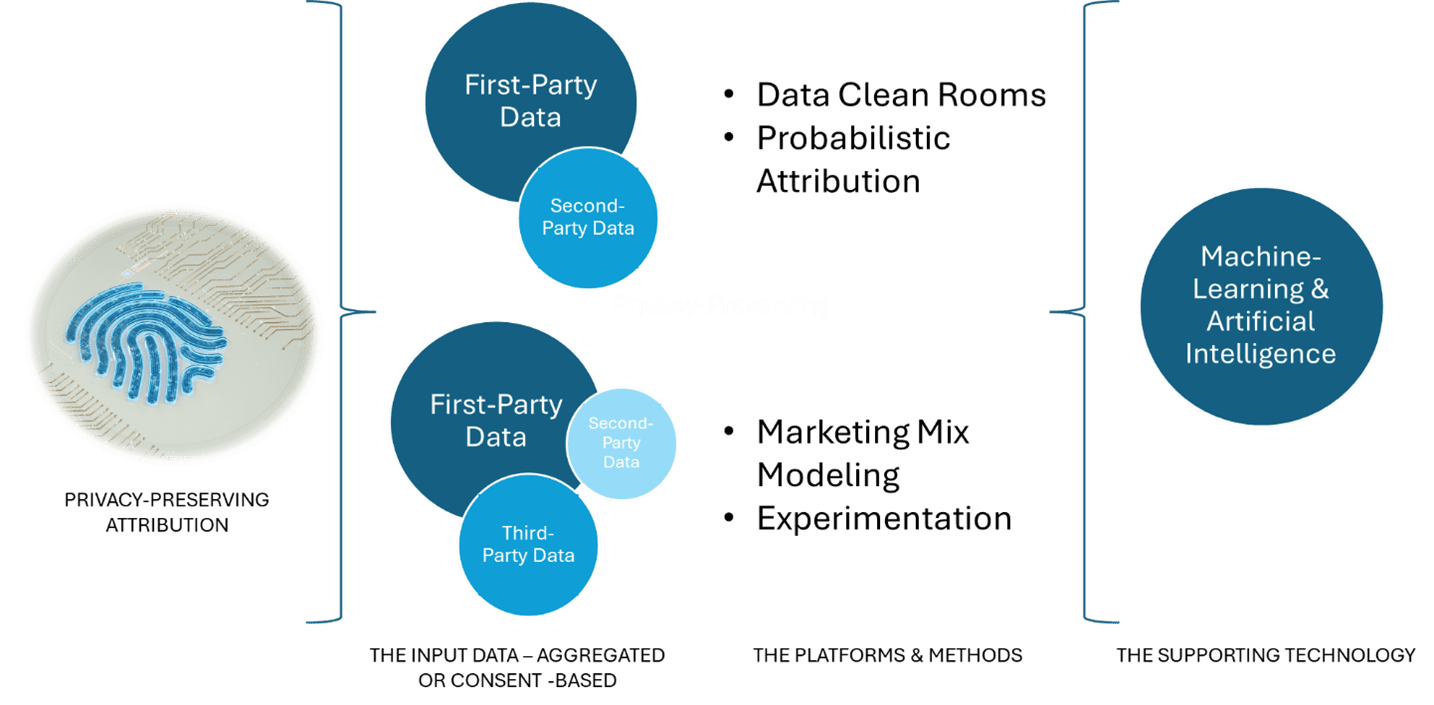
First-Party Data Takes Center Stage: Marketers are rediscovering the power of first-party data – information they collect directly from customers. Research indicates that 85% of marketers consider first-party data essential to their strategy[2]. By leveraging website behavior, app interactions, and purchase history, they can create a rich data set that exposes how different marketing touchpoints (emails, social media ads, banners, etc.) influence conversions. Building strong customer relationships and obtaining explicit consent for data collection will be crucial for companies to be able to gather and use this type of data for marketing analytics and targeting.
Privacy-Preserving Technologies Are Essential: In the wake of increased privacy regulations and consumer demand for data transparency, MTA providers have embraced privacy-first approaches. Indeed, a recent study found that customers are more likely to buy from companies that are transparent about how their data is used[3]. From differential privacy techniques to anonymized data aggregation, these strategies ensure that sensitive information is protected while still delivering actionable insights to marketers. Contextual targeting has also regained renewed importance as a powerful marketing tool. By placing ads based on the content of the web page being viewed rather than tracked user information, contextual targeting can still deliver personalized and effective messages, and valuable insights into campaign performance, while respecting user privacy.
Data Clean Rooms Emerge as a Collaboration Hub: Data clean rooms offer a secure environment where multiple parties can combine their first-party data sets without revealing any underlying personal information. By leveraging data clean rooms, companies can conduct sophisticated attribution analysis, perform audience matching, and measure campaign effectiveness with enhanced precision. For example, a retailer can upload anonymized transaction data along with marketing touchpoint data into a clean room, which is then securely matched with anonymized audience segments from a publisher. The system can then analyze the combined data through advanced algorithms to attribute transactions to specific marketing channels, providing insights into conversion paths, channel effectiveness, and audience segmentation. This approach not only ensures data privacy but also fosters collaboration between brands, publishers, and platforms, leading to richer, more actionable marketing insights. There are two broad categories of data clean room providers:
- Walled Gardens: Offered by tech giants (Google, Meta, Amazon), they boast vast internal amounts of data (depth) but limit external data sources (breadth).
- Pure-Play Providers: Independent companies (e.g. Snowflake, Habu, InfoSum) focus on secure collaboration and data processing tools, offering flexibility to connect various external data sources (breadth) but potentially less pre-existing data (depth) compared to walled gardens.
While data clean rooms offer powerful insights into campaign performance while respecting user privacy, they are often very expensive, complex and not real-time (so of limited use for some channels such as programmatic). The world’s largest companies are doing some testing & learning in this space, but it is still a very young and constantly evolving technology and democratization might still be some way ahead.
Focus on Probabilistic Attribution: As reliance on third-party cookies shifts away, there's a significant movement from deterministic to probabilistic modeling. Deterministic models, which depend on precise data points and exact user matches, are becoming less feasible in a privacy-conscious world where data is often anonymized and fragmented. In contrast, probabilistic modeling leverages advanced statistical techniques, such as Bayesian modeling, and machine learning algorithms to make educated guesses about user behavior and identity across different touchpoints. For example, they might look at the timing and frequency of ad exposures relative to conversions to determine probable influence. By analyzing patterns and correlations within large datasets, probabilistic models can effectively fill in the gaps left by the absence of direct user identifiers, while offering a flexible and robust approach to understanding and predicting customer journeys.
MMM is Back with Faster and Deeper Insights: With Marketing Mix Modeling (MMM) making a comeback given the data challenges faced by traditional MTA solutions, modern MMM solutions can provide marketers with the granular and high-frequency answers they are looking for, in a privacy-compliant way. Using granular, high-frequency, high-recency data, a solution such as Campaign Response Attribution (CRA) offers marketers a credible alternative to MTA while seamlessly blending into a total Return On Marketing Investment (ROMI) framework. Whereas traditional MTA focuses on cookie level data over very short time windows, CRA can provide similar learnings across both off and online through higher frequency (hourly), time series data, without the need for any PII (Personal Identifiable Information). For a large asset management firm, the ML-powered solution used priors from MMM developed with daily data in the last three years augmented by hourly data for the last three month in order to provide the latest insights on granular campaign performance across channels, partners, day parts, creatives, ad length, search keywords etc., while accounting for external factors. The solution thereby offers direction on how to optimize granular tactics and messages for greater conversion, within the broader ROMI context of strategic investments guidance. In essence, it extends the holistic learnings from MMM to more granular and frequent insights.
Incrementality Experimentation as an Add-On: Another technique that has seen renewed attention is incrementality testing. Rather than simply attributing conversions to specific touchpoints, incrementality tests seek to quantify the incremental impact of marketing efforts by comparing outcomes from a test group exposed to a campaign with a control group that isn't. This allows marketers to identify the additional conversions or sales generated by the campaign itself. Proponents argue that experiments can be used in tandem with Marketing Mix Modeling (MMM) to calibrate and refine those models by comparing outputs of MMMs and experiments and ensure that they broadly concur. In more sophisticated instances, outputs can be used as priors in the MMM when Bayesian modeling is employed, or to define a valid range for the model coefficient estimates, in the case of other modeling techniques. However, incrementality tests have a number of drawbacks and flaws that marketers need to be aware of:
- Complexity and Cost: Setting up and running incrementality tests can be complex and resource intensive. It may require specialized expertise and involve running multiple campaigns simultaneously, which can be challenging.
- Time Commitment: Results can take time to gather as sufficient data needs to be collected from both test and control groups. This can be a drawback for marketers seeking quick insights.
- Limited Scope: As a result of complexity, tests typically focus on a single campaign or channel at a time, making it difficult to assess the overall effectiveness of a complex marketing mix and therefore limiting the ability to use these results as priors in MMM.
- External factors: non-controllable events such as competitor actions or seasonal events can influence consumer behavior and skew the results.
- Point In Time Specific: Tests happen at specific points in time which leads to knowledge gaps and prevents continuous monitoring of performance.
- Budget Waste: A certain number of control impressions need to be bought with placebo advertising which reflects lost media investments.
Machine Learning and AI Power Attribution: One of the most exciting developments in marketing attribution is the integration of Machine Learning (ML) and Artificial Intelligence. These technologies have empowered marketers to rapidly analyze vast amounts of data and uncover complex patterns even from fragmented datasets. They enhance attribution models by using multi-touch and algorithmic approaches for more accurate credit assignment. While data privacy is a concern, AI tools can achieve identity resolution and cross-device tracking through probabilistic matching. This allows marketers to build unified customer profiles and gain a comprehensive understanding of the customer journey. Their real-time automation features in terms of data ingestion and processing, variable transformations and preliminary model selection help produce faster insights. For true causality inference however, additional statistical techniques need to be used such as highlighted in our blog. The predictive nature of AI-based tools also supports the budget optimization process by running through thousands of media response curves in seconds and simulating the impact of various marketing scenarios on business KPIs. Personalization is enhanced as technology such as Generative AI can analyze contextual and behavioral signals to identify customer preferences. This allows businesses to create customer journey maps and granular customer segments, leading to more effective marketing campaigns and product development. Leading retailers like Amazon and Walmart have been using AI to provide personalized product recommendations to their customers and increase sales[4].
Overall, the evolution of Machine Learning and AI has equipped marketers with comprehensive consumer insights without compromising privacy or relying on traditional tracking mechanisms.
The New Era of Attribution: A Shift in Mindset
The decreasing trackability of the digital landscape isn't just about technology; it's about a change in mindset. Marketers and marketing leaders need to move towards a future where attribution is less about pinpointing the exact touchpoint that led to a conversion, and more about understanding the overall customer journey and optimizing campaigns for long-term success. This is particularly important as the CMO has become a growth catalyst for the organization, strategically supporting all other C-suite functions in the achievement of sustained business growth, as highlighted in a recent study[5]. As the steward of first-party data investments, the CMO can not only inform the development of targeted messaging campaigns but also provide actionable feedback to product teams. As such, harnessing first party-data empowers the CMO to identify emerging customer needs and preferences, which in turn can catalyze innovative product development and enhance overall customer satisfaction.
Embracing the Future: A Brighter Outlook for Attribution
As we look ahead, the future of attribution is bright with possibilities. Advancements in data analytics, machine learning, and privacy technologies will continue to drive innovation in attribution modeling. By focusing on building strong customer relationships, harvesting the resulting first party data, and adopting new attribution models, marketers can emerge stronger and more adaptable in the privacy-first era. The future of multi-touch attribution may be different, but it's far from bleak. It's a chance for the industry to build a more privacy-focused, integrated, and holistic approach to measuring marketing effectiveness, positioning businesses for long-term success.