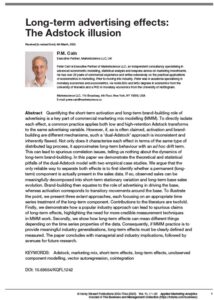
Long-term advertising effects: the Adstock illusion
2025 | | Journal Articles
Quantifying both the short-term activation and long-term brand-building effects of advertising is a central part of commercial marketing mix modelling (MMM). In this paper, we demonstrate both theoretically and empirically how the popular ‘dual Adstock’ approach leads to spurious claims of long-term effects. Not only does this highlight the need for more credible measurement techniques, it shows how ‘the long-term’ needs to be clearly defined and categorised if commercial MMM practice is to provide meaningful industry generalisations.
Long-term advertising effects: the Adstock illusion
Quantifying both the short-term activation and long-term brand-building effects of advertising is a central part of commercial marketing mix modelling (MMM). To directly isolate each effect, a common practice includes both short and high-retention Adstock variables in the estimating equation. However, if, as is often claimed, activation and brand-building are different mechanisms, such a ‘dual-Adstock’ approach is inconsistent and inherently flawed. Not only does it characterize each effect in terms of the same type of distributed lag process, it approximates long-term advertising effects with an ad hoc drift term. This can lead to spurious correlation issues and tells us nothing about the dynamics of long-term brand-building.
In this paper we demonstrate the theoretical and statistical pitfalls of the dual-Adstock model with two empirical case studies. We argue that the only credible way to separate short-term activation and long-term brand building effects is to first identify whether a permanent (long-term) component is actually present in the sales data. If so, observed sales can be meaningfully decomposed into short-term variation and long-term (base) evolution. Brand-building effects then equate to the impact of advertising on base evolution, whereas activation effects correspond to transitory variation around the baseline. To illustrate the point, we survey three extant approaches, each focusing on an appropriate time series treatment of the long-term component.
Contributions to the marketing literature are twofold. Firstly, we demonstrate both theoretically and empirically how a popular industry approach can lead to spurious claims of long-term effects, highlighting the need for more credible measurement techniques in practical MMM work. Secondly, we show how long-term effects can mean different things depending on the time series properties of the data. Consequently, if commercial MMM practice is to provide meaningful industry generalisations, long-term advertising effects need to be clearly defined and categorised. We conclude with managerial implications followed by avenues for future research.
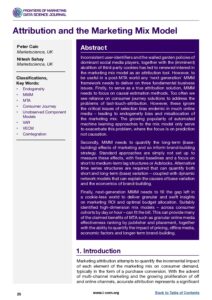
Attribution and the Marketing Mix Model
2023 | FRONTIERS OF MARKETING DATA SCIENCE JOURNAL, I-COM | Journal Articles
Marketing Mix Modeling (MMM) has seen a resurgence as a marketing attribution tool since the recent setbacks suffered by Multi-Touch Attribution (MTA) due to stricter data privacy regulations and the imminent abolition of third-party cookies. However, to be useful in a post-MTA world, any ‘next generation’ MMM framework needs to deliver on three fundamental business issues. This paper explores these issues and offers practical solutions.
Attribution and the Marketing Mix Model
Inconsistent user-identifiers and the walled garden policies of dominant social media players, together with the (imminent) abolition of third-party cookies has led to renewed interest in the marketing mix model as an attribution tool. However, to be useful in a post-MTA world any ‘next generation’ MMM framework needs to deliver on three fundamental business issues.
Firstly, to serve as a true attribution solution, MMM needs to focus on causal estimation methods. Too often we see reliance on consumer journey solutions to address the problems of last-touch attribution. However, these ignore the critical issues of selection bias endemic in much online media – leading to endogeneity bias and misallocation of the marketing mix. The growing popularity of automated machine learning approaches to the mix model only serve to exacerbate this problem, where the focus is on prediction not causation.
Secondly, MMM needs to quantify the long-term (base building) effects of marketing and so inform brand-building strategy. Standard approaches are simply not set up to measure these effects, with fixed baselines and a focus on short to medium-term lag structures or Adstocks. Alternative time series structures are required that can quantify both short and long-term (base) variation – coupled with dynamic network models that can explain the causes of base variation and the economics of brand-building.
Finally, next-generation MMM needs to fill the gap left in a cookie-less world to deliver granular and swift insights on marketing ROI and optimal budget allocation. Suitably identified high dimension mix models – across consumer cohorts by day or hour – can fit the bill. This can provide many of the claimed benefits of MTA such as granular online media effectiveness ranking by publisher and placement with the added benefit of quantifying the contribution of pricing and offline media, controlling for the wider economic environment and the ability to analyze brand building.
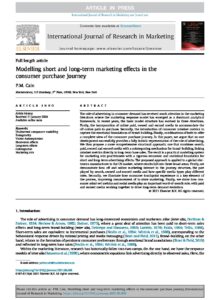
Modelling short and long-term effects in the consumer purchase journey
2021 | International Journal of Research in Marketing | Journal Articles
In this paper, we propose a comprehensive structural approach to the modern mix model: one that combines search, paid, owned and earned media with a cointegrating mechanism for brand-building, linking mindset metrics directly to long-term base sales. The result is a practical modelling system for marketing mix practitioners with a rigorous economic and statistical foundation for short and long-term advertising effects.
Modelling short and long-term effects in the consumer purchase journey
The role of advertising in consumer demand has received much attention in the marketing literature, where the marketing response model has emerged as a dominant analytical framework. In recent years, the basic model structure has evolved in three directions. Firstly, the incorporation of online paid, owned and earned media to accommodate the off-online path-to-purchase. Secondly, the introduction of consumer mindset metrics to capture the emotional foundations of brand-building. Finally, combinations of both to offer a complete view of the consumer purchase journey. In this paper, we argue that no one development successfully provides a fully holistic representation of the role of advertising. We thus propose a more comprehensive structural approach: one that combines search, paid, owned and earned media with a cointegrating mechanism for brand-building, linking mindset metrics directly to long term base sales. The result is a practical modelling system for marketing mix practitioners with a rigorous economic and statistical foundation for short and long-term advertising effects. The proposed approach is applied to a global electronics manufacturer in the US market, where results fall into three broad areas. Firstly, we demonstrate how off and online marketing interact in the journey structure, the part played by search, owned and earned media and how specific media types play different roles. Secondly, we illustrate how consumer touchpoint experience is a key element of the process, improving measurement of in-store marketing. Finally, we show how consumer mind-set metrics and social media play an important word-of-mouth role, with paid and earned media working together to drive long-term demand evolution.
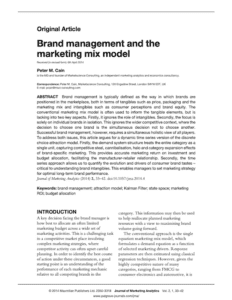
Brand management and the marketing mix model
2014 | Journal of Marketing Analytics | Journal Articles
Successful brand management requires a simultaneous holistic view of all players in the market. This paper argues for a dynamic time series version of the discrete choice attraction model, where the entire category is treated as a single unit. The resultant model leads to more accurate estimates of competitive steal, cannibalisation, halo and category expansion effects of brand specific marketing.
Brand management and the marketing mix model
Brand management is typically defined as the way in which brands are positioned in the marketplace, both in terms of tangibles such as price, packaging and the marketing mix and intangibles such as consumer perceptions and brand equity. The conventional marketing mix model is often used to inform the tangible elements, but is lacking into two key aspects. Firstly, it ignores the role of intangibles. Secondly, the focus is solely on individual brands in isolation. This ignores the wider competitive context, where the decision to choose one brand is the simultaneous decision not to choose another. Successful brand management, however, requires a simultaneous holistic view of all players.
To address both issues, this paper argues for a dynamic time series version of the discrete choice attraction model. Firstly, the demand system structure treats the entire category as a single unit, capturing competitive steal, cannibalisation, halo and category expansion effects of brand specific marketing. This provides accurate marketing ROI and budget allocation, facilitating the manufacturer-retailer relationship. Secondly, the time series approach allows us to quantify the evolution and drivers of consumer brand tastes – critical to understanding brand intangibles. This enables managers to set marketing strategy for optimal long-term brand performance.
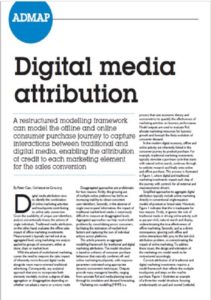
Dynamic MMM and Digital Attribution
2014 | Admap | Journal Articles
This article presents an aggregate modelling framework for traditional and digital marketing attribution. The model structure is based on a theory of consumer purchase behaviour that naturally combines off and online marketing touchpoints. Outputs provide many managerial benefits, ranging from accurate ROI and media planning inputs through to simulation and demand forecasting.
Dynamic MMM and Digital Attribution
Digital media attribution aims to identify the combination of online marketing activities and touchpoints contributing to online sales conversion. Given the availability of unique user-identifiers, analysis conventionally traces the actions of single individuals. Traditional media attribution, on the other hand, evaluates the offline sales impact of offline marketing investments. Measurement is typically carried out at an aggregated level, using marketing mix analysis applied to groups of consumers, either at store, chain or market level. With the advent of multi-channel marketing, comes the need to measure the sales impact of inherently micro-focused digital media alongside more macro-oriented traditional advertising. Consequently, any analytical approach that aims to incorporate both elements inevitably involves a degree of data aggregation or disaggregation depending on whether we adopt a macro or a micro route.
This article presents an aggregate modelling framework for traditional and digital marketing attribution. The model structure is based on a theory of consumer purchase behaviour that naturally combines off and online marketing touchpoints, with response parameters estimated using appropriate dynamic econometric techniques. Outputs provide many managerial benefits, ranging from accurate ROI and media planning inputs through to simulation and demand forecasting.
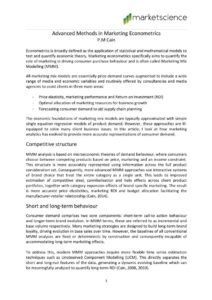
Advanced Methods in Marketing Econometrics
2014 | | White Papers
This article outlines three broad innovations in MMM techniques ranging from competitive structure and the measurement of long-term effects through to incorporation of digital and social media. All such innovations lead to increased flexibility and realism with more accurate client deliverables, helping to maintain the relevance of MMM in the modern digital economy.
Advanced Methods in Marketing Econometrics
Econometrics is broadly defined as the application of statistical and mathematical models to test and quantify economic theory. Marketing econometrics specifically aims to quantify the role of marketing in driving consumer purchase behaviour and is often called Marketing Mix Modelling (MMM). All marketing mix models are essentially price demand curves augmented to include a wide range of media and economic variables and routinely offered by consultancies and media agencies to assist clients in three main areas:
1. Price elasticity, marketing performance and ROI
2. Optimal allocation of marketing resources
3. Forecasting consumer demand to aid supply chain planning
The economic foundations of marketing mix models are typically approximated with simple single-equation regression models of product demand. However, these approaches are ill-equipped to solve many client business issues. In this article, we look at how marketing analytics has evolved to provide more accurate representations of consumer demand.
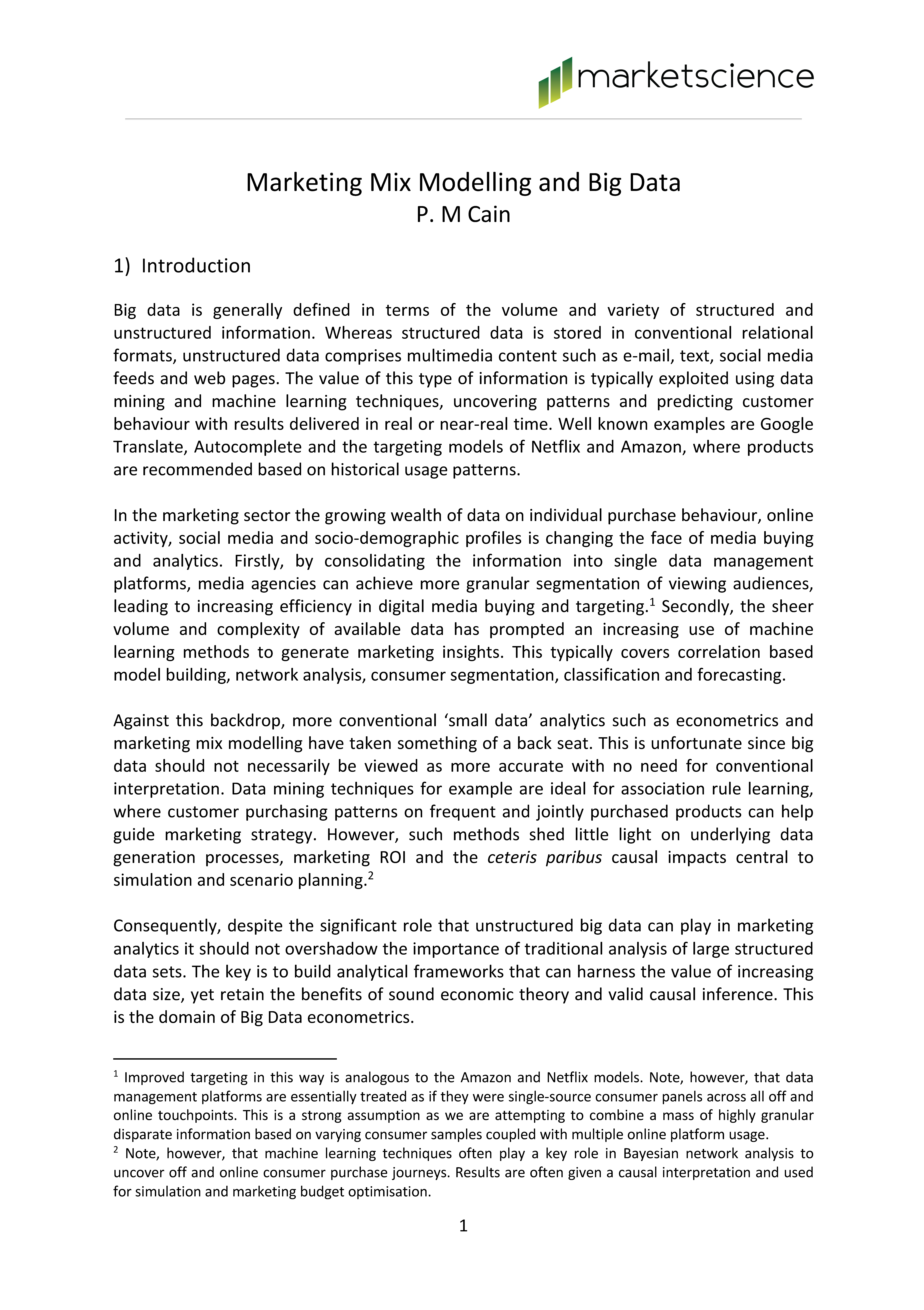
Marketing Mix Modeling & Big Data
2014 | | White Papers
In the wake of the Big Data revolution, analytical methods such as data mining and machine learning have taken center-stage in unstructured data analysis. Although such methods can play a role in marketing analytics, it is important not to lose sight of the distinct advantages of ‘small’ structured data methods such as econometrics which play a key role in marketing ROI, simulation and causal based inference.
Marketing Mix Modeling & Big Data
In the marketing sector the growing wealth of data on individual purchase behavior, online activity, social media and socio-demographic profiles is changing the face of media buying and analytics. Firstly, by consolidating the information into single data management platforms, media agencies can achieve more granular segmentation of viewing audiences, leading to increasing efficiency in digital media buying and targeting. Secondly, the sheer volume and complexity of available data has prompted an increasing use of machine learning methods to generate marketing insights. This typically covers correlation-based model building, network analysis, consumer segmentation, classification and forecasting.
Against this backdrop, more conventional ‘small data’ analytics such as econometrics and marketing mix modelling have taken something of a back seat. This is unfortunate since big data should not necessarily be viewed as more accurate with no need for conventional interpretation. Data mining techniques for example are ideal for association rule learning, where customer purchasing patterns on frequent and jointly purchased products can help guide marketing strategy. However, such methods shed little light on underlying data generation processes, marketing ROI and the causal impacts central to simulation and scenario planning. Consequently, despite the significant role that unstructured big data can play in marketing analytics it should not overshadow the importance of traditional analysis of large structured data sets. The key is to build analytical frameworks that can harness the value of increasing data size, yet retain the benefits of sound economic theory and valid causal inference. This is the domain of Big Data econometrics.
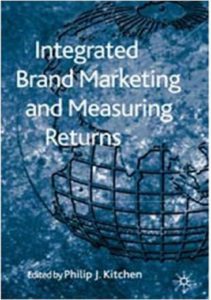
Marketing mix modelling and return on investment
2010 | Integrated Brand Marketing and Measuring Returns, Palgrave Macmillan | Journal Articles
This article provides an overview of the commercial marketing mix model (MMM), from economic foundations, through statistical estimation to commercial outputs.
Marketing mix modelling and return on investment
This article provides an overview of the commercial marketing mix model (MMM), from economic foundations, through statistical estimation to commercial outputs. Against the background of the conventional approach, we put forward alternative theoretical and econometric frameworks for improved short-term ROI evaluation, together with techniques for evaluating the long-term effects of marketing investments and how these may be combined with short-term results to provide total ROI. We conclude with a discussion of the managerial benefits of the mix model and the total returns on marketing.
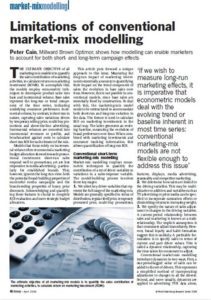
Limitations of Conventional Marketing Mix Modelling
2008 | Admap | Journal Articles
This paper puts forward an alternative approach to marketing mix modelling which explicitly models both the short and long-run features of the data. Not only does this provide more accurate short-run marketing results but, when combined with evolution in intermediate brand perception measures, allows an evaluation of the long-run impact of marketing activities.
Limitations of Conventional Marketing Mix Modelling
The primary function of all marketing mix models is to quantify the sales contribution of marketing activities, with a view to calculating Return on Investment (ROI). To accomplish this, all such models employ econometric techniques to decompose product sales into base and incremental volume. Models that focus solely on incremental volume often recommend a marketing budget allocation skewed towards promotional activity: short-run sales respond well to promotions, yet are less responsive to media activity – particularly for established brands. This, however, ignores the long-run view: that is, the potential brand-building properties of successful media campaigns on the one hand and the brand-eroding properties of heavy price discounts on the other. Acknowledging and quantifying these features is crucial to a complete ROI evaluation and a more strategic budget allocation.
This article puts forward a unique approach to resolving this issue. Measuring the long-run impact of marketing investments essentially amounts to quantifying their impact on the trend component of the sales series: that is, on the evolution in base sales over time. However, this is not possible in conventional models since base sales are essentially fixed by construction. To deal with this problem, the marketing mix model needs to be re-structured as an Unobserved Component Model (UCM) to accommodate both short-run and long-run variation in the data. The former is used to calculate ROI on marketing investments in the usual way. The latter measures the evolution of brand preferences over time. This generates an evolving baseline which, when combined with marketing investments and consumer tracking information, allows a quantification of long-run ROI.