Marketscience’s Peter Cain recently published an Editorial piece in the 4th Issue of I-COM’s Frontiers of Marketing – Data Science Journal. Peter provides a point-of-view around the application of AI in the Marketing Mix Modeling context and suggests caution in using it as a tool for causal analysis and parameter identification.
To read the full paper, click here:
Below is the Editorial piece:
Marketing attribution aims to quantify the incremental contribution of each element of the marketing mix on the consumer path to purchase, typically in the form of a sales conversion. Outputs form the basis of ROI calculations and optimal budget allocations, helping to inform marketing strategy and the planning cycle.
Attribution measurement approaches generally fall into three camps: disaggregated Multi-Touch Attribution (MTA), aggregated Marketing Mix Modeling (MMM) and (unified) combinations of the two. However, given concerns surrounding user-identifiers, walled gardens, GDPR, third-party cookies and overestimation of short-term performance media, MTA is now rapidly falling out of favor. This has seen a resurgence in the popularity of MMM, notably amongst key players such as Google and Facebook.
Whereas it is certainly true that aggregated MMM solutions can overcome many of the privacy concerns of MTA, bias towards short-term performance marketing remains a core problem. Yes, the time series dimension can help redress the balance between short term activation and long-term brand-building. However, like MTA, the selection bias inherent in much online media confounds correlation and causation, where (part of) the 'treatment' outcome (sales) is caused by a factor that predicts the likelihood of selection into treatment rather than the treatment itself. For example, consumers with a greater propensity to buy predicts the level of search traffic, which in turn predicts the sales outcome. As such, a large proportion of site visits are simply an artefact of the sales process. This creates an endogeneity or identification problem, leading to biased estimates of the search-sales impact and all marketing effects that work through it.
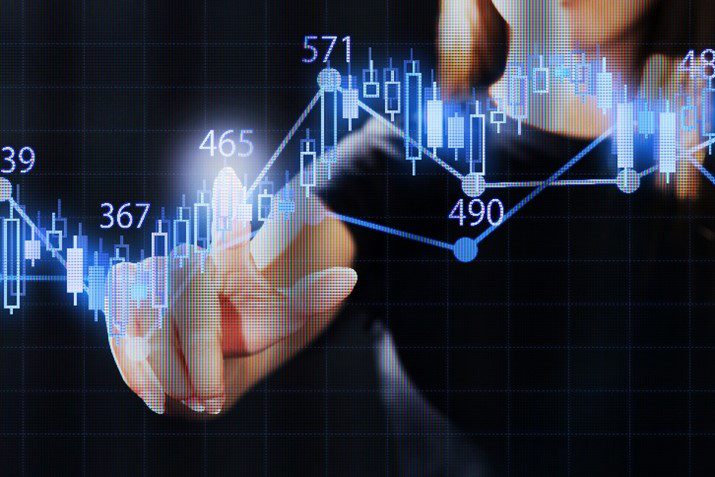
The growing popularity of Al and automated machine learning (ML) techniques has only exacerbated this problem, where rigorous causal analysis is sacrificed for the promise of rapid 'real-time' MMM delivery at scale. This is fine if the goal is simply a set of best-fitting predictive models: after all, stepwise regression has been around for a long time. However, if the aim is to uncover the 'structural' cause and effect relationships necessary for accurate budget allocation, standard Al/ ML approaches simply don't cut it. This has led to the introduction of 'causal' Al techniques, which attempt to discover the causal pathways present in an observational data set, based on Directed Acyclic Graph (DAG) structures (inter alia, Pearl, 2000). However, since human context is always required, such techniques 'do not yet work as stand-alone methods for causal learning' (Peters et al, 2017). Furthermore, there is rarely one unique chain: endogeneity bias stems from ignoring the simultaneous likelihood of all plausible DAGs in the data. Consequently, Al techniques are useful for uncovering sets of competing (causal) chains but cannot automatically solve the selection bias problem per se.
So what is the way forward? One popular solution lies in experimental design research such as A/B testing. Advocates contend that this is the only way to assess true incrementality. Results can then act as Bayesian priors to provide some form of 'ground truth' in both conventional and Al-driven MMM solutions, thereby solving the selection bias problem. However, even setting aside objections to Randomised Controlled Trials as the gold standard of causal inference (inter alia, Deaton and Cartright, 2018), experimentation results are rarely available as part of the MMM data collection process. Even if they were, experiments would need to be run across all endogenous variables at considerable expense: an impractical solution in the vast majority of cases.
In practice, therefore, we generally have to do the best we can with observational data and seek recourse in more traditional parameter identification techniques: namely, statistical methods designed to separate correlation from causation such that the estimated parameter reflects a true incremental effect. Candidates range from Instrumental Variables (IV), Difference in Differences, regression discontinuity designs and Heckman correction factors, through to Latent IV, Gaussian Copulas and weighted DAG analysis. Whichever route is taken, the message is clear: for meaningful attribution, the chosen identification scheme needs to be clearly specified as part of any MMM engagement. This requires careful human judgement. No amount of automated Al bluster can escape this central fact.
To learn more about how Marketscience can help you with all your marketing attribution needs, please contact us!
References:
- Deaton, A. and Cartwright, N. (2018). "Understanding and misunderstanding randomized controlled trials", Socia/ Science & Medicine (210), Pages 2-21.
- Pearl, J. (2000), "Causality", New York: Cambridge University Press.
- Peters, J., Janzing, D., & Scholkopf, B. (2017). "Elements of Causal Inference: Foundations and Learning Algorithms" (Adaptive Computation and Machine Learning series). The MIT Press.