Multi Touch Attribution Solved
Alternative to MTA with detailed feedback of digital marketing programs
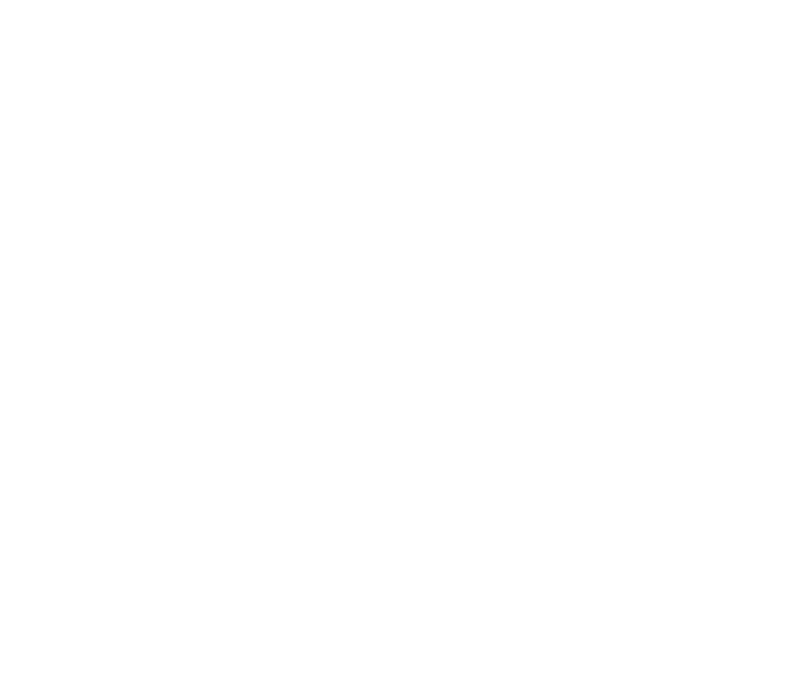
Campaign Response Attribution (CRA) provides marketers with a credible alternative to Multi Touch Attribution (MTA). CRA is a real-time solution measuring the performance of live campaigns providing direction on how to optimize marketing tactics and messages for greater conversion. CRA measures which elements of a brand's marketing efforts are working harder to drive response and offers a better currency than last-touch attribution for trading in any response-based media from traditional DR TV, through CTV and OTT to traditional Display and Search.
CRA is a complement to MMM, the gold standard for measuring true incrementality and ROI, by offering a real-time solution to improve Marketing Effectiveness.
Pending cookie deprecation and stricter privacy controls are limiting the ability of traditional multi touch attribution models to measure response based on individual click streams and 3rd party data. CRA offers a solution based on the most granular data available using reports at greater time frequencies and based on cohort cookie groups.
Digital response media drives need for MTA solutions
With the advent of multi-channel marketing, comes the need to measure the sales impact of inherently micro-focused digital media alongside more macro-oriented traditional advertising. Consequently, any analytical approach that aims to incorporate both elements inevitably involves a degree of data aggregation or disaggregation depending on whether we adopt a macro or a micro route.
In response, modern marketing analytics has evolved into two broad strands: Network Models and Multi Touch Attribution.
In this approach, customer journey theories attempt to provide a fuller explanation of the final purchase decision with paid, owned and earned media working together to drive demand. Measurement is typically carried out at an aggregated level, using econometric methods applied to groups of consumers, either at store, chain or market level. Outputs are used to quantify ROI, advise on optimal budget allocation across off and online channels and produce sales forecasts.
Network approaches to short and long-term MMM lie at the heart of our BaseDynamics system.
Multi touch attribution (MTA) is a micro analogue of short-term MMM – focusing on a network of interactions between online media on the road to purchase. Measurement is based on the online journeys of single individuals using various techniques ranging from econometric choice modelling (Logit) and probability chains (Markov) through to economic game theory (Shapely values). The result is a final (average) sales equation where each online touchpoint is free of any last-touch bias.
Outputs allow the marketer to assign credit to each touchpoint and to dig deeper into the digital mix, addressing more precise tactical questions such as how, where and when to spend the allocated budget. Specifically which networks, publishers, messages and keywords are driving the greatest response.
Challenges implementing MTA
A recent survey and article by MMA outlines some of the key challenges companies have faced implementing an MTA solution. They identify challenges in Data, Methodology and Organization. The Data challenge is clear with the walled gardens already blocking a common cookie, the now pending total deprecation by Google and the already implemented revisions to IDFA by Apple. The combination of these actions renders MTA a moot point at least in terms of "respondent level" analysis. No longer are we able to create a 'click-stream' connecting on site transactions with third party ad placements. Identity solutions are a hot topic but still seem far off.
At the same time, MTA faces three more theoretical challenges:
MTA analysis is typically carried out using ‘tall’ panel data sets: namely, a large number of high-frequency cookie-level records observed over relatively short time periods. As such, the time-series properties of the data cannot be captured and results are short-term focused
The causal impact of each last-touch variable is questionable. MTA just controls for all observable paths between marketing touchpoints but does not fully control for reverse causality between those touchpoints and sales. Consequently, unless the level of disaggregation can isolate pre-disposed/non-pre-disposed customers - via separation into new and existing for example - simultaneity bias is still present.
MTA is a very narrow, micro representation of consumer demand. It has no control for pricing, offline media and economic factors routinely incorporated into more macro MMM studies. This problem has led to an increasing desire over recent years to combine MTA with MMM into a Unified Marketing Impacts Model (Forrester, 2015). These approaches are dubiously defined as “a blend of statistical techniques that assigns business value to each element of the marketing mix at both a strategic and tactical level.”
Conventional industry solutions
The marketing measurement industry has developed two main approaches to overcoming the limitations of traditional Multi Touch Attribution models. These approaches look to leverage the benefits of MMM but in an ad hoc and limited way.
Unified models aim to combine MTA and MMM data streams into one unified data set. This needs to overcome three key challenges
- To maintain the granularity of the cookie level data, a unified data set would entail relatively ad hoc division of the MMM input data into higher (daily/hourly) frequencies.
- Matching the market level data to the relevant cookie-level cohorts.
- Even if a well-matched data set of sufficient length is possible, the market-level variables are still aggregated concepts. As such, their effects will typically be under-estimated in the high variance environment of the online cookie data.
Given these problems, a more palatable approach is a two-step method that uses the outputs from one as inputs into the other. This gives two options.
1. MMM outputs as priors in MTA
Online media parameter estimates, split by traffic source such as display, search, email and affiliate, enter as priors into Multi Touch Attribution to constrain the total estimated impact. MTA can then assign credit to the components of each aggregated bucket. The main advantage of this approach is that the MMM estimates can be treated as meaningful priors because:
- We have full control for all offline and external factors, together with time series dimension.
- With a baseline and a suitable identification strategy, the MMM parameters satisfy the Conditional Independence Assumption and can thus be treated as causal effects.
The question is then how best to introduce the priors into the MTA analysis so we maintain consistency between incremental effects of MTA and MMM. The obvious solution is to apply a constant incrementality fraction to all conversion paths. However, this is subject to two key problems:
- Biased conversion credit for the component parts of the relevant touchpoint.
- Control for the offline and non-addressable effects along each conversion path – introducing aggregated effects/data into the micro model.
2. MTA outputs as priors in MMM
This approach requires all the granular online data streams be fed into the MMM analysis – aggregated to weekly level – with estimated MTA coefficients as priors. There are two main problems here:
- Heavily parameterised and subject to a high degree of collinearity
- MTA coefficients are misleading as priors since:
- With no baseline or identification strategy, MTA is not a genuine causal framework but simply a variable importance analysis. Higher frequency cookie level data – leading to lagging – or displacement in time - does not solve the endogeneity/simultaneity problem.
- No control for offline and economic factors
A preferable unified approach that solves these issues is as follows:
- Identify the parameters of an MTA using latent instrumental variable techniques
- Create compound indices for MTA parameters that summarise the causal incremental across component parts and consumer groups (DMA, region for example).
- Use A/B testing to estimate the unobserved confounding 'walled garden' effects of Facebook, AdWords, Instagram, Pinterest and YouTube.
- Create compound indices across component parts and consumer groups (DMA, region for example).
- Roll up all indices to weekly level and use as regressors alongside traditional offline media and pricing in aggregated MMM
- Impose suitable priors on parameters to reflect MTA results
Commercial mix models claim to move beyond MMM and MTA, involving more holistic models of the business at increased levels of disaggregation covering regions, stores, channels and individuals.
Whereas greater disaggregation is indeed critical to capture heterogeneity of consumer demand, this approach is nothing new in the industry: with standard Hierarchical Bayesian techniques, such highly disaggregated modelling is commonplace – with insights regularly provided across markets, brands, products and customers. Coupled with dynamic time-series estimation and network approaches to the off-online consumer decision journey, modern MMM analysis can already provide a comprehensive view of the client business and offers more aggregated alternatives to conventional MTA analysis.
To provide anything new, advances in MMM analysis need to address the endogeneity at the heart of causal analysis. Only then can such advances really claim to provide a true picture of the clients’ business and genuine incrementality of marketing investments. Increased disaggregation can sometimes achieve this – where fixed effect longitudinal modelling can help sweep away any unobserved heterogeneity. However, this is certainly no guarantee and alternative solutions are typically required such as (latent) instrumental variables and/or network techniques. Cost and time permitting, such techniques can also be supplemented with quasi-experimental A/B testing to quantify causal effects of walled-garden data.
The Marketscience Solution
Campaign Response Attribution (CRA) takes the most granular level data available during the campaign window and builds decision tree based models to create predictive response models. The impression data available is typically at a higher time frequency, by hour/minute versus daily/weekly, and at a customer cohort level. We marry this to 1st party customer sales data that is typically at a timestamp level and create 'tall' (hourly, cohort), 'short' (1 to 3 months) longitudinal panels. This more granular data over a short time interval lends itself to decision tree based modeling approaches as there is reduced need to control for any underlaying dynamics associated with time-series analysis.
In this way, Campaign Response Attribution can provide a suitable replacement for Multi Touch Attribution with additional benefits that allow us to:
- Rank online media effectiveness at most granular level (by publisher, by placement etc)
- Incorporate offline media
- Assess synergies between online media.
- Incorporate the time dimension
CRA estimates a tall, high frequency panel model, using:
- Hourly/minute level data
- High disaggregation over consumers groups (store/household/cohort)
- Base and seasonal priors estimated from an auxiliary aggregated dynamic time-series panel model
In a 'Short-Interval' context there are limited longer-term time-series dynamics at play. Any base shifting or changes in seasonality are minimal when looking at a short timeframe. As such we can utilize more efficient algorithms to estimate the relationships in the data.
A simple approach is the traditional linear regression. However more efficient decision tree models or gradient boosting algortithms are able to parse through data faster and assess more automated transformations and segmentation of results. Integration of 'priors' on expected relationships is possible and ensures consistency.
Models are built using training data sets and validated on test datasets. Overfitting is reduced by using randomized hold out samples in model development and final models are estimated using an ensemble technique.
Marketscience is not a one-size-fits-all analytics solution
Independent. Critical. Objective
No one specific model is the be-all-end-all of marketing attribution. We believe better insights come from improved model-building - with particular attention paid to the quantification of causal relationships wherever possible.
That's why we build dynamic mix models that reflect our clients' unique marketing ecosystem, industry, and environment. Each solution is designed to bring data science to the center of decision-making in ways that best fit your organizational workflow.
To help achieve this, we offer a combination of consultancy, software solutions, and training academies.
Putting Your Marketing Data To Work
It's not always easy to see direct, causal relationships between your company's marketing initiatives and sales conversions.
MTA has long promised but largely failed to deliver meaningful insights on true effectiveness and ROI of marketing campaigns. CRA overcomes the barriers to traditional MTA and side-steps challenges of current privacy standards to deliver on the original promise of MTA and inform campaign mamagment with realtime insights to help increase marketing effectiveness.
Boost transparency in your marketing mix decision-making process based on the latest, most relevant marketing science. To get the guidance you need to achieve improved statistical analysis and optimize for more impactful marketing initiatives contact Marketscience today.