Marketing Mix Modeling
Comprehensive Measurement of Marketing Effectiveness
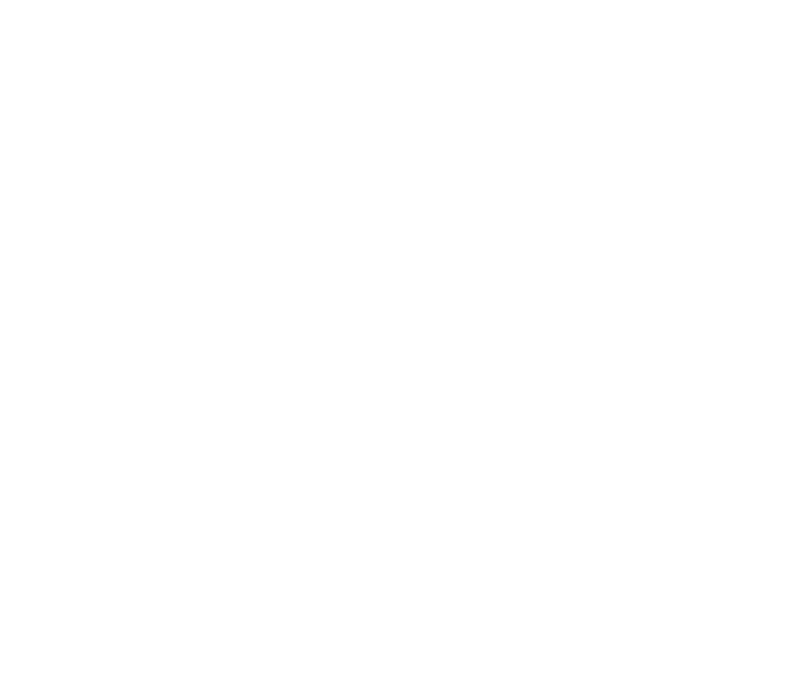
BaseDynamics is an innovative Marketing Mix Modeling (MMM) framework based on network models of short-term sales growth and long-term brand building. This unique MMM solution provides marketers with the most complete and accurate measurement of marketing's impact on business results.
Improves on the conventional Marketing Mix Model with:
1. An explicit treatment of long-term brand building effects
2. Control for the simultaneity bias inherent in last-touch performance media
3. Greater depth of analysis with Dynamic Hierarchical Bayesian estimation
Creating better marketing budget optimization based on:
1. A more realistic measurement of marketing's total impact on sales
2. The true relative order of marketing channel effectiveness
3. Insights for product positioning and creative development
What is marketing mix modeling?
Marketing mix modeling is a process that uses economic theory and econometrics to quantify the effectiveness of marketing efforts on company sales and business performance.
The Marketing Mix Model
The marketing mix model is a widely-used tool to evaluate Return on Investment (ROI) and inform optimal allocation of the marketing budget. Economics and econometrics lie at the heart of the process.
In the first place, the model structure is derived from microeconomic theories of consumer demand ranging from single equations of product sales to full interactive systems of brand choice.
Secondly Ordinary or Generalized Least Squares regression techniques are typically used to measure the impact of various marketing variables, including other independent factors like economic indicators, on a dependent variable such as sales or website traffic.
The model is used to quantify the impact of marketing investments on sales and other desired business outcomes. This then informs managers and senior decision-makers across marketing, sales and finance how best to allocate marketing spend and other investments to drive business strategy.
The conventional marketing mix modeling process
In today's digital economy, online and offline activity are inherently linked in the consumer journey to product purchase. For example, traditional paid offline TV advertising may stimulate a purchase cycle that starts with natural online search, continue through to owned website research - involving price checking and feature comparisons - leading to targeted social media messaging and earned recommendations in private social channels, before finally ending in a purchase online or instore. Read More.
Correct attribution of traditional and digital marketing requires a model framework that reflects the multiple paid, owned and earned media touchpoints and intermediate steps on the road to purchase as illustrated in Figure 1.
Figure 1: The Conventional Marketing mix model ecosystem
The selected marketing mix variables need to represent the full range of marketing programs as well as the other relevant drivers of intermediate and final business outcomes.
Drivers typically encompass various marketing activities such as pricing, sales promotions, TV, radio, print ads, display, search, direct mail marketing campaigns, seasonality, macroeconomic factors and extreme events.
Marketing variable transformations are applied based on how sales and other journey steps are expected to relate to the independent driver variables. These may be multiplicative, yielding constant elasticity demand functions, or additive linear models where response elasticities depend on the level of sales and drivers. Further transformations can also be specified to incorporate saturation or diminishing return effects. Ultimately the model estimation process identifies which transformations best fit the data and explain the business outcomes.
To fully quantify the impact of marketing investments, the mix model needs to capture not only the current-period impacts on business outcomes but also the carry-over' effects associated with successful advertising campaigns: namely, where the effects are felt beyond the period of execution due to product purchase cycles and/or media retention in the mind of the consumer. To capture these 'dynamic' relationships, AdStock or Polynomial Distributed Lag transformations can be applied. Furthermore, given factors such as brand loyalty and habit formation, additional dynamic specifications can also be incorporated to properly account for sales persistence over time.
The final modeling step quantifies the demand response to variation in each of the driver variables and is typically based on multivariate econometric techniques, designed to separate product sales into base and incremental volumes. Incremental volumes are the typical focus of the standard mix model and essentially short-run in nature, capturing the week-to-week transitory variation in sales driven by each of the driver variables. Base sales, on the other hand, represent the long-run or trend component of the product time series.
Incremental volumes are converted into incremental revenues or profits and benchmarked against costs to calculate ROI to each element of the marketing mix. This forms the basis of regular reports for marketing and senior managers. Model response parameters are then fed into optimization and simulation tools, allowing marketers to optimize investments and maximize desired business outcomes.
Two key problems with the conventional Marketing Mix Model
The conventional model ignores the inherent selection bias of many forms of online media leading to the continued over-attribution to last touch acquisition marketing channels.
The conventional MMM ecosystem approach is designed primarily to deal with the problem of last-touch attribution - handled via a nested sequence of equations mimicking the consumer journey to sales. The objective is to reattribute a part of last-touch media back to the original source earlier in the chain.
However, this focuses on just one predetermined causal flow. It ignores the inherent selection-bias of last-touch media such as paid search, where consumers with the highest propensity to buy will be the most likely to take part in the paid search 'treatment'.
Such latent purchase predisposition implies that a part of paid search is simply an artefact of the sales process and we are essentially regressing an element of sales on itself. As such, sales and search could equally well be reversed in the chain, leading to a simultaneity or endogeneity problem.
Without accounting for the likelihood of this type of chain-reversal, the sales impact of paid search predominantly captures simple correlations rather than meaningful causal effects. This leads to biased estimates of the search-sales impact and all marketing effects that work through it. Consequently, even if offline TV advertising does lead to more branded search activity, it does not necessarily mean it drives incremental sales in this way.
The conventional model is short-term focused by construction and fails to address the drivers of long-term sales evolution including the mechanics of brand-building.
To fully describe sales patterns, marketing mix models need to reflect both short and long-term marketing effects. Short-term effects explain mean-reverting or transitory sales variation, whereas long-term effects explain persistent or permanent changes in underlying base sales.
Standard models focus solely on short-term sales effects with Adstock transforms and fixed or deterministic baselines. However, this fails to reflect any persistent changes in core brand preferences. As such, potential long-term brand-building impacts of marketing are precluded by definition.
Conventional approaches attempt to incorporate long-term effects as simple extensions of the short-term structure.
- Longer AdStocks: advertising data are adstocked with very high retention rates, indicative of heavy persistence of past levels of advertising weight.
- Scaling factors: long-run effects are derived simply by multiplying the short-term effects by an appropriate scaling factor.
- Attitudinal brand metrics: survey-based 'mindset' metrics are included as regressors in the model, together with sub-models in terms of advertising variables. The indirect effects of advertising on sales via these mindset metrics are then interpreted as long-term effects.
Longer AdStocks simply extend the 'mean-reverting' standard dynamic approach, whereas arbitrary scaling factors are ad hoc, bearing no relation to the data at hand.
The use of brand perception metrics is often the most common approach. However, simply adding these data as additional regressors in short-term sales models fails to represent the long-term structure. In order to provide a meaningful foundation for long-term effects, brand perception data need to:
- Relate directly to long-term base sales: base sales and mindset metrics are alternative measures of underlying brand health and two-sides of the same coin. The use of observed sales is ad hoc and obscures long-term movements, risking contamination with short-term transactional effects.
- Explain any evolution in (base) sales: if attitudinal data are to provide a meaningful long-term foundation, they need to explain the base sales evolution inherent in genuine brand-building: that is, share a common trend with sales and cointegrate to form equilibrium relationships. However, sales evolution is ignored and the impact of advertising on mindset metrics is deemed to reflect brand-building behavior per se.
- Provide a meaningful brand-building mechanism: simply including a brand perception metric as a regressor does not mimic the brand-building process. Namely, how base sales, mindset metrics and earned media (word of mouth) interact and drive each other leading to long-term brand-effects that wear in or accrue over time.
BaseDynamics: the Marketscience approach
To address these issues, the Marketscience approach takes an alternative more holistic approach to the marketing mix model, separating demand generation into two linked networks:
- a short-term off-online consumer journey controlling for selection bias
- a long-term brand-building journey, where the long-term preferences embodied in attitudinal data are causally linked to long-term preferences reflected in base sales
Figure 2: Short-term sales and long-term brand building networks
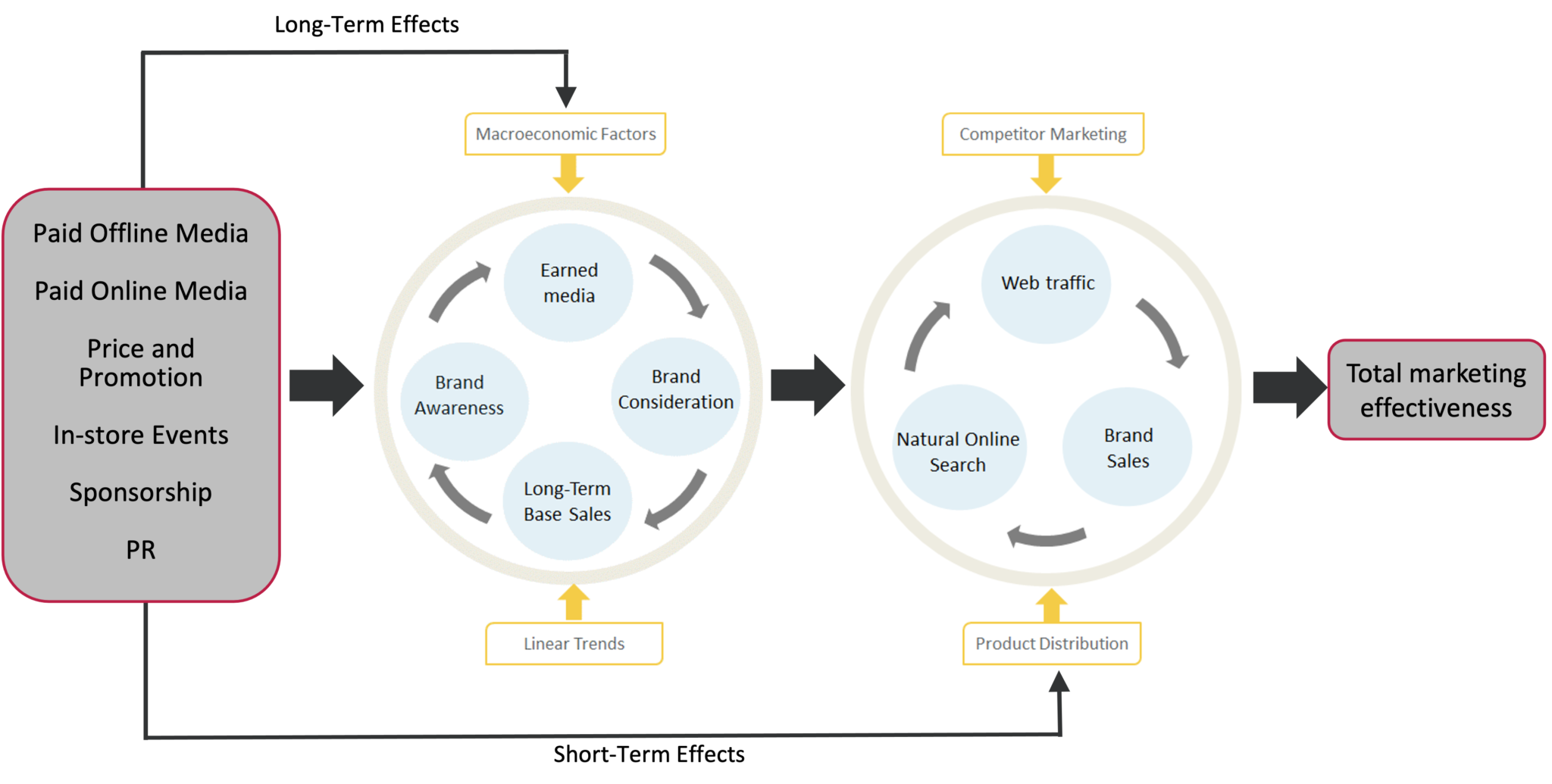
The combination of both networks fully captures the impact of short-term marketing and long-term brand-building and estimated with a unique combination of time-series techniques.
The short-term network
A dynamic unobserved components model (UCM)
- A more flexible form of the conventional approach, separating observed sales into dynamic baseline, seasonal, cyclical, regression effects and random error
- Direct estimation of short-term response parameters and lag structures (AdStocks)
- Nested sub models to control for last click bias
- Network modeling or IV to control for simultaneity and the multiple potential paths to purchase
- Controls for and extracts long-term evolution
The long-term network
A brand-building Vector Error Correction Model
- Network brand-building model combining base sales with brand metrics and other consumer attitudinal data
- Incorporates long-term cointegrating relationships between base sales, brand metrics, earned media, word of mouth and other journey steps
- Long-term preferences embodied in attitudinal data are causally linked to long-term preferences reflected in base sales
- Attitudinal mindset metrics provide a credible structural foundation for the brand-building effects of advertising
A worked example of the BaseDynamics approach
We start with an Unobserved Component Model using daily sales data including:
- Dynamic base
- Dynamic daily seasonal components
- Off and online marketing (with lags)
- Promotions
- Covid summary and outliers
Figure 1: General form of Unobserved Component Model:
Figure 2: Example UCM Output extracting the Trend
Additional models, analysis and outputs for the short-term network included:
- Sub-equations for all endogenous variables
- DAG analysis for endogeneity control
- Decompositions and short-term ROI
Step 1: model of the long-term dynamic system
Figure 1: General VAR(X) model form
Figure 2: Model data
Step 2: Test the economic structure and identify true long-term impact
- Statistical testing for long-term cointegrating relationships between the variables
- Economic structure shows one long-term equilibrium cointegrating vector (CV1) between base sales, price, awareness and new business starts
- Feedback between base sales and awareness shows how awareness both drives base and adjusts to word of mouth and product performance/experience
- Impulse response reflects feedback and delivers true long-term impact of awareness on base
- Provides more credible estimates of marketing effects that work through long-term brand metrics
Step 3: identify brand building structure and wear-in
- Vector error correction model (VECM) quantifies dynamic adjustment of each network variable
- Long-term TV and social media effects work through unaided brand awareness onto base sales (wear-in)
- Long-term elasticities equal to awareness elasticity weighted by base-awareness elasticity (0.12)
Figure 3: Impulse response functions
Step 4: Model decomposition and long-term ROI calculation
- Base sales decomposition deliver long-term sales volumes over model sample and beyond
- Combined with NPV calculation of average weekly base volumes over a two-year forecast horizon
How does the Marketscience approach help?
With the advent of digital media, the marketing response model has evolved to capture a modern paid, owned, earned media structure. The conventional approach takes the form of a nested sequence of regression equations, where paid marketing works through intermediate own and earned media touchpoints to drive sales/business outcomes. Such a ‘consumer-journey’ structure is analogous to an aggregated form of Multi Touch Attribution and designed primarily to deal with last-touch attribution problems where a part of a last-touch medium such as paid search is re-attributed back to sources earlier in the chain such as TV advertising. Read More
However, nested sequences like this still assume a given causal chain and ignore the inherent selection bias of online media such as paid search. This occurs when a (part of) the impact of the paid search ‘treatment’ on sales is caused by a (latent) factor that predicts the likelihood of selection into treatment rather than due to the treatment itself. As a result, simple regressions of sales on search clicks – a component of site visits – are inherently biased since those consumers with the highest propensity to buy are more likely to take part in the paid search ‘treatment’.
Such latent purchase predisposition implies that paid search tends to act more as a distribution channel and represents an artefact of the sales process. As such, we are essentially regressing a part of sales on itself. The upshot is that sales and search could equally well be reversed in the chain, leading to a simultaneity or endogeneity problem, where the sales impact of paid search predominantly captures simple correlations rather than meaningful causal effects. Read More
This leads to biased estimates of the search-sales impact and all marketing effects that work through it. Consequently, even if offline TV advertising does lead to more branded search activity, it does not necessarily mean it drives incremental sales in this way.
Only by correctly adjusting for simultaneity bias in the consumer journey structure will we get accurate measures of the relative and absolute performance of marketing channels and correct recommendations for optimal budget allocation and maximization of marketing ROI. See Case Study
Pricing plays a central role in consumer demand analysis across all sectors of the economy, from consumer packaged goods, airlines, technology and entertainment through to telco and financial services. A key challenge is how best to set prices for optimal sales and brand performance. More specific pricing questions include:
- What is my optimal short-term discount level?
- How can I set base or msrp prices to minimize brand equity erosion and maximise profits?
- How can I set pricing dynamically over time to optimize sales and revenue targets?
- How do I best estimate brand, UPC and SKU level price response for products and brands?
- What is the optimal interest rate strategy for new and existing customers?
These questions are best addressed as part of the BaseDynamics MMM approach as it is able to measure base and promo prices in the appropriate long and short term models and so is better able to estimate the appropriate elasticities. See Case Study
The depth of advice depends on the granularity of analysis: At high levels of aggregation, pricing response is more contaminated by distributional effects and price is more of a general control factor. At lower levels - such as sales channel, product and store level - (dynamic) price elasticities are more actionable for optimal price-setting.
Unobserved Component Model approaches to the marketing mix model provide the ability to measure genuine dynamic baseline evolution. We are then able to quantify the drivers of base evolution in a VAR/VECM framework based on identified cointegrating relationships between base sales, brand metrics and earned media. The result is a more credible structural foundation for the mechanics of persistent brand-building - lost in models that simply include brand perception metrics as additional regressors in the standard mix model. Read More
Cointegration looks at how the variables are tied together based on common trends and represents a stronger relationship than simple correlation since the variables co-evolve and the relative distance between them is constant, indicative of an underlying attractor relationship. Within this framework we are able to evaluate the brand-building role of a broad range of mindset metrics: from more general metrics such as Awareness and Consideration to more specific metrics that might apply in a given category such as Provides a Great Experience or Is a Trusted Partner. By evaluating these metrics and their impact on sales we can inform strategies for optimal product positioning and creative development. See Case Study
Competitor actions are typically included as explanatory variables in the marketing mix model, with hypothesised negative impacts on sales. However, it is often preferable to model these effects in the context of the entire category.
Single-equation MMM forms are often applied in practice to several competing products in one group or ‘category’. However, sets of single equations are unrelated to each other and do not ‘add-up’, telling us nothing about genuine category effects of product marketing. To overcome this problem, simultaneous equation demand system approaches are required. With these types of models we can see if a company’s marketing or the marketing of its competitors leads to changes in market share, total category sales or both. Read More
If marketing serves to shift market share between companies, we might expect to see both short and long term aspects resulting in either temporary share shifts and/or more permanent changes in category structure.
In order to analyse these types of dynamic interaction we have extended the short and long term network model to incorporate simultaneous equation demand system approaches.
The UCM approach to MMM breaks sales time series into short and long-term components - providing a natural sales forecasting framework. The estimated model time series structure can then be extrapolated forwards and combined with planned media strategies and forecasts of other drivers to provide more accurate sales forecasts and so improve supply chain planning and expectation setting.
Read More: IJRM 2005, IJRM 2021.
Marketscience is not a one-size-fits-all analytics solution
Independent. Critical. Objective
No one specific model is the be-all-end-all of marketing attribution. We believe better insights come from improved model-building - with particular attention paid to the quantification of causal relationships wherever possible.
That's why we build dynamic mix models that reflect our clients' unique marketing ecosystem, industry, and environment, where each solution is designed to bring data science to the center of decision-making in ways that best fit your organizational workflow.
To help achieve this, we offer a combination of consultancy, software solutions, and training academies.
Putting Your Marketing Data To Work
It's not always easy to see direct, causal relationships between your company's marketing initiatives and sales conversions.
Marketing mix modeling is a popular solution, exploiting economic theory and econometrics to quantify the effectiveness of marketing activities on sales and business performance. However, traditional static approaches are ill-equipped to successfully quantify short and long-term marketing effectiveness.
BaseDynamics represents an innovative approach, designed to encompass the economic foundations of both short and long-term effects based on our latest research. The result is actionable insights helping you plan marketing strategies, deploy smarter marketing tactics and build brand equity.
Boost transparency in your marketing mix decision-making process based on the latest, most relevant marketing science. To get the guidance you need to achieve improved statistical analysis and optimize for more impactful marketing initiatives contact Marketscience today.